- Change theme
The Machine Learning Models Detecting Collusion in Online Poker Tournaments
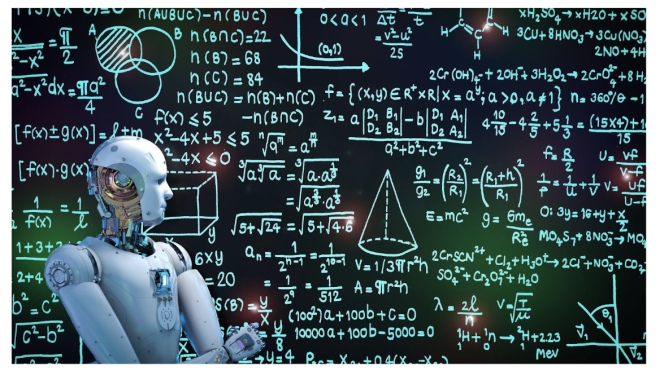
Around the dark areas of online poker a mental fight ensues between cheating players and detection systems designed by programmers.
04:12 07 May 2025
Around the dark areas of online poker a mental fight ensues between cheating players and detection systems designed by programmers. Millions in digital card room transactions occur daily but artificial intelligence models run detection scans for hidden patterns beyond human observation abilities. Artificial monitoring systems act as fascinating convergences between game theory and behavioral analytics together with artificial intelligence to reshape security controls across virtual poker platforms.
The Hidden Threat in Virtual Card Rooms
Online poker tournaments received widespread popularity in the last ten years because they unite players who want to play Texas Holdem and experience exciting poker games worldwide. Online platforms maintain high vulnerability because players cannot interact directly with one another. Online gambling sites currently depend on technology to spot cheating operations because their managers lack traditional floor observation methods for spotting suspicious body language or strange actions during face-to-face games.
Poker collusion manifests through various methods which include basic chip dumping actions and strategic information sharing about hole cards and coordinated betting sequences between players. Prominent poker tournaments present attractive financial prizes that extend to million-dollar prize pools. The extreme combination between secret identities and significant financial rewards establishes ideal conditions for people who want to cheat.
How AI Learns to Spot the Cheaters
Anti-collusion systems in modern times combine several machine learning models that operate together to detect fraud. Statistical anomalies detected in gameplay data constitute the initial layer which also looks for unusual player table meeting frequencies as well as highly unusual win rates and suspicious timing patterns.
The examination of massive hand data enables the system to create lawful player conduct standards through millions of processed hands. The algorithms utilize their defined parameters to mark gameplay behaviors which deviate from standard norms. When two players meet at identical tables in excessive numbers which exceeds statistical norms it creates obvious suspicious activity.
Such advanced models focus on conducting comprehensive assessment of betting behavior patterns. The system analyzes the mutual player reactions to determine any possible indications of knowledge transfer between participants. Through observation across hundreds of tournaments the AI detects consistent folding from Player A during aggressive moves of Player B before confirmed observations of strong hands by Player B.
These systems prove effective because they demonstrate learning and adapting skills. Neural networks in modern collusion detection systems use advanced learning algorithms to consistently develop their understanding of suspicious behavior patterns. This process supersedes early methods which deployed rigid rule-based systems. The AI adjusts its detection approaches to match the developments made by colluding players.
Beyond Simple Pattern Recognition
Anti-collusion systems that exist at the highest level use both game theory algorithms and psychological modeling procedures. The software examines player decisions to determine if their actions mirror what logical thinking would produce when a player exposes themselves to the available information.
The network process analyzes betting sequences to understand how an uncertain large bet decision by a player could only make sense through knowing their opponent's cards unless they possess outside information about opponent holdings. These models evaluate whether experienced poker players would naturally use this decision without accessing illegal information.
Current gaming platforms monitor players through biometric metrics and tracking methods and subtle movement data along with decision durations which produce one-of-a-kind identification profiles for each gamer. Such systems detect whether various accounts belong to the same person which identifies a technique that collusion schemes commonly use.
The Arms Race Continues
Modern technological innovations have not eliminated the difficulty of detecting deceptive behavior. Cheating operations evolve due to their skilled nature by implementing advanced encryption protocols that pair with time management method changes and refined signaling protocols for eluding monitoring systems. Machine-learning methods are used by some users to discover hidden weaknesses in detection software.
The situation resembles a standard security competition where each party develops new defenses against the other side. Online poker operators need to enforce detection measures without creating false accusations that might wrongly penalize innocent operators. Research groups work steadily to create advanced detection methods which catch even the most secretive forms of collusion.
In Closing
The fight to stop collusion in online poker functions as one of the most interesting uses of machine learning in gaming security. These systems converted a substantial poker weakness into controlled risks that made possible the expansion of online poker into a legitimate competitive market.
AI systems and human behavioral patterns work together in a complex manner to advance the possible limits of upholding game rules even though absolute game security remains impossible. Machine learning models continue to develop sophistication which enables them to anticipate new techniques of collusion and prevent these attempts from harming the fundamental fairness in competitive poker.